Understanding the Importance of Data Annotation for Modern Businesses
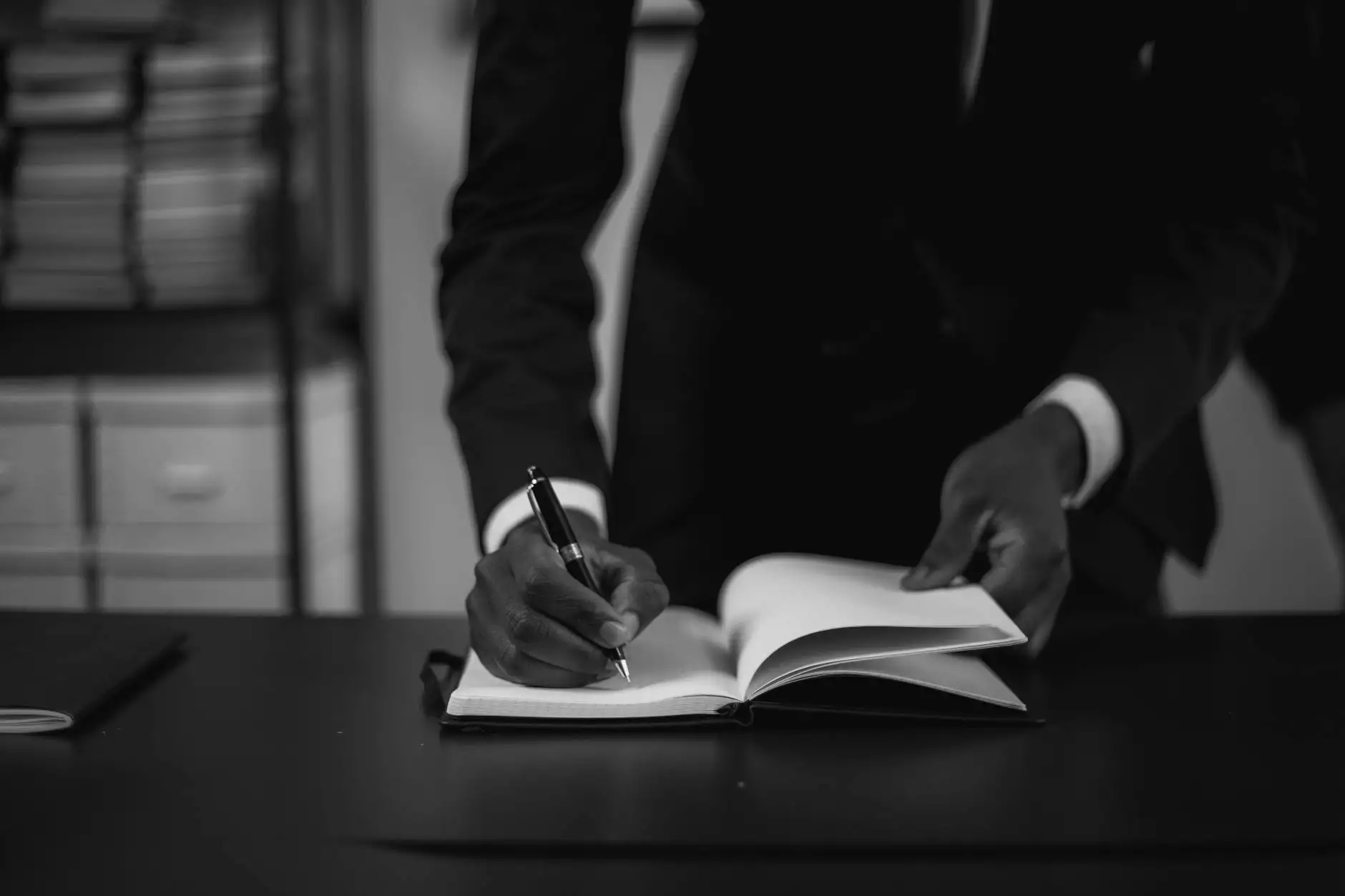
In the age of artificial intelligence (AI) and machine learning, the term data annotation has emerged as a cornerstone for businesses seeking to leverage technology. As companies increasingly rely on data-driven decision-making, the demand for accurate and effective data annotation continues to grow. This article will explore what data annotation is, its significance in various industries, and how platforms like KeyLabs.ai are revolutionizing the data annotation landscape.
What is Data Annotation?
Data annotation is the process of labeling data to make it understandable for machine learning algorithms. This involves attaching meaningful tags or metadata to different types of data, such as images, text, audio, and video. By doing so, we help algorithms recognize patterns, classify data, and learn from input examples.
The Types of Data Annotation
Data annotation encompasses various forms of data, each with its unique requirements. Here’s a detailed look at the primary types:
- Image Annotation: Involves labeling images with relevant tags or bounding boxes to identify objects, actions, or attributes. Common applications include self-driving cars and medical imaging.
- Text Annotation: This includes tagging parts of speech, identifying entities, and annotating sentiments within textual data. It's crucial for natural language processing (NLP) applications.
- Audio Annotation: Involves adding labels to sound clips; it's essential for speech recognition algorithms and can help in identifying different sounds or noises.
- Video Annotation: Combines elements of image and audio annotation to label video frames with specific actions or events, greatly used in surveillance and sports analytics.
The Role of Data Annotation in Industries
The significance of data annotation extends across numerous industries, showcasing its versatility and necessity. Here are a few key sectors that benefit immensely from high-quality data annotation:
1. Healthcare
In healthcare, annotated medical images are used for diagnostics, predicting diseases, and even in research to enhance patient care. Accurate imaging annotation is crucial for developing AI-driven software that can assist radiologists in identifying abnormalities.
2. Automotive
The automotive industry relies heavily on data annotation to develop autonomous driving systems. Annotated data sets help self-driving cars understand complex environments, recognize traffic signs, pedestrians, and other vehicles.
3. Marketing
In marketing, text annotation allows companies to analyze customer sentiments, feedback, and trends in consumer behavior. This information is essential for creating targeted marketing strategies.
4. Retail
Retail businesses use annotated data to optimize inventory management and enhance customer experience through personalized recommendations. Image annotation helps in sorting products visually and enhancing online shopping experiences.
5. Security and Surveillance
Data annotation is critical in surveillance for identifying suspicious activities. By annotating video data, security systems can learn to distinguish between normal and abnormal behavior.
Challenges in Data Annotation
While data annotation is essential, it is not without its challenges. Some of the common issues include:
- Scalability: As businesses grow, the volume of data increases exponentially, making it harder to annotate data at scale without compromising quality.
- Quality Assurance: Ensuring high-quality annotations is critical. Inaccurate labeling can lead to misinformed AI decisions.
- Cost: Data annotation can be a time-consuming and expensive process, especially when done manually.
- Consistency: Maintaining consistency in how data is annotated across different teams and projects is essential for reliable outcomes.
How to Overcome Data Annotation Challenges
To tackle these challenges effectively, businesses need to adopt strategic approaches:
1. Using Data Annotation Tools
Platforms like KeyLabs.ai offer comprehensive data annotation tools that automate many aspects of the process, ensuring speed and efficiency. These tools often come with built-in quality assurance mechanisms that help maintain high standards.
2. Implementing a Hybrid Approach
Combining automated and manual annotation can help achieve a balance between speed and quality. For instance, initial annotations can be done through AI tools, which are then validated and refined by human annotators.
3. Continuous Training and Feedback
To ensure consistency and quality, continuous training programs for annotation teams are vital. Regular feedback loops can help improve processes and refine annotation techniques.
The Future of Data Annotation
As AI technology continues to advance, the future of data annotation looks promising. Here are some anticipated trends:
- Increased Use of AI for Annotation: Tools leveraging AI will become more sophisticated, allowing for faster and more accurate annotations.
- Growing Need for Multi-Modal Annotation: The need for annotating various data types in tandem (like text with images) will rise, especially for complex AI models.
- Demand for Real-Time Annotation: Industries such as automotive and healthcare will require real-time data annotation capabilities to keep pace with rapid technological advancements.
- Focus on Ethical Considerations: As AI impacts more areas of life, ethical data annotation practices will become increasingly vital to ensure fairness and transparency.
Conclusion
In conclusion, data annotation stands at the forefront of AI and machine learning developments across various sectors. Companies that invest in accurate and efficient data annotation platforms, such as KeyLabs.ai, will have a significant competitive edge in the data-driven era. By overcoming the challenges associated with data annotation, businesses can maximize the potential of their data, leading to innovation, improved customer experiences, and enhanced operational efficiency.
As we look to the future, embracing the advancements in data annotation and understanding its critical role will be vital for any business aiming to thrive in a fast-evolving technological landscape.